SONATAnotes
How IT Departments Can Make Peace with Generative AI
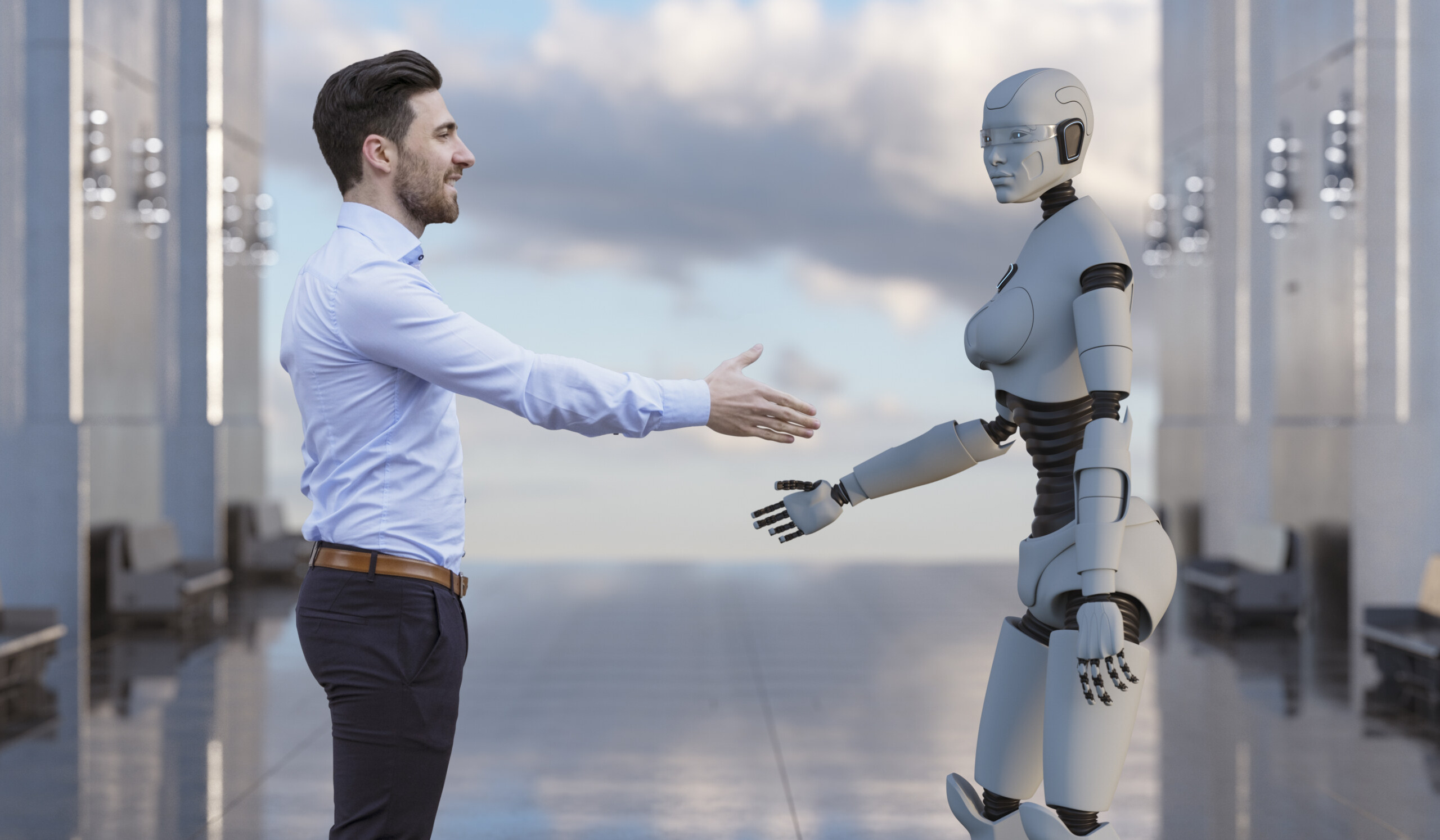
These days, everyone from the marketing department to R&D to finance has been swept up in the mania around AI platforms like ChatGPT, Claude, and Gemini, and are looking for any reason to start using AI at work. However, for IT departments, generative AI presents a host of unknown risks and liabilities, comparable to when organizations first started migrating from on-premise systems to cloud apps.
So how are IT professionals to respond when business stakeholders ask (or demand… or don’t even ask) to use AI tools at work? How can we assess and control the risks and set sensible policies when the technology itself is evolving at breakneck speed from month to month and quarter to quarter?
In this article, we’ll look at the reasons why IT departments might consider allowing generative AI in the workplace, the stances your department can take towards AI, for how to assess the risks and benefits, and the pros and cons of different policies for specific organizations.
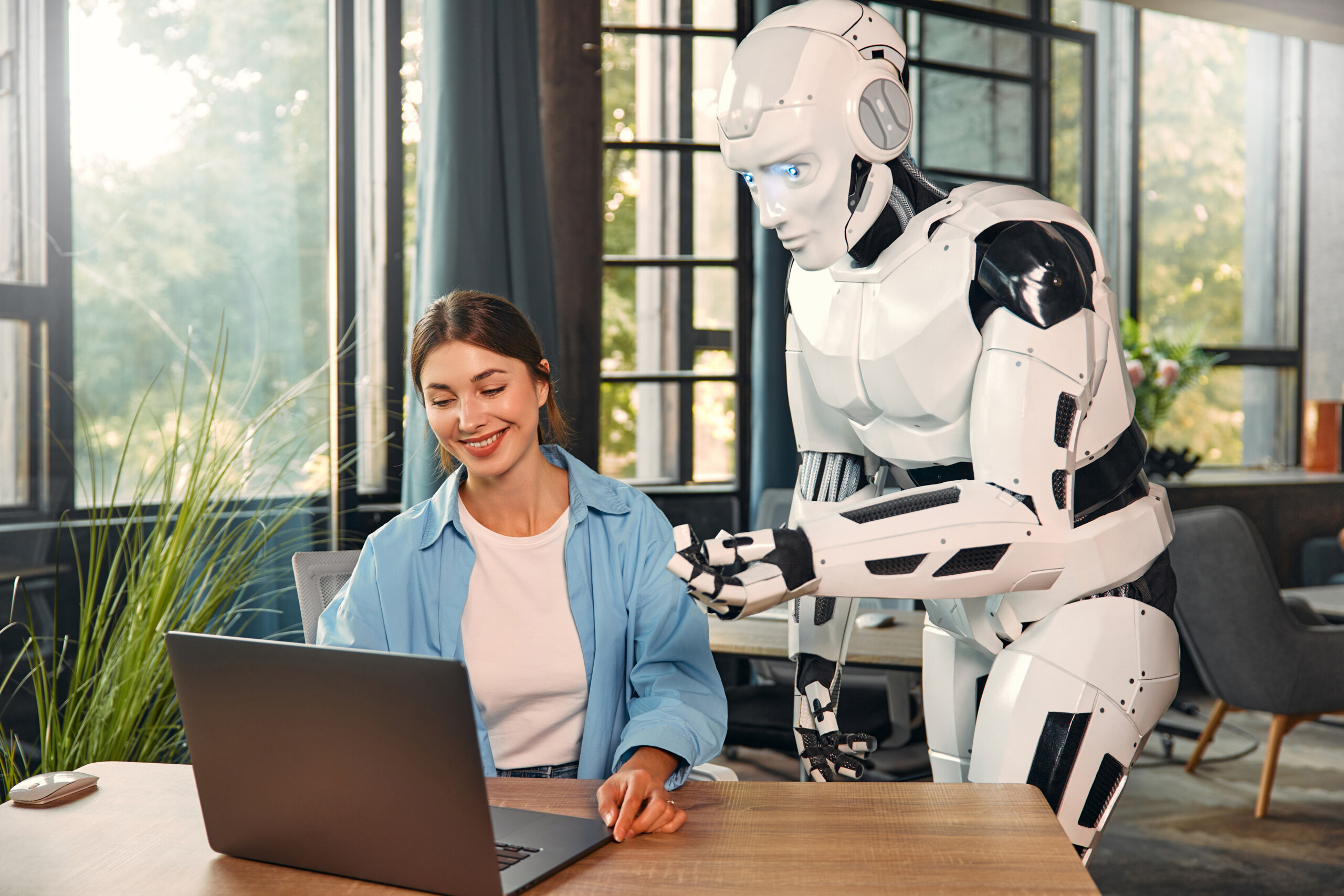
Benefits of AI in the Workplace
Before we dive into the complexities of regulating AI use, it might be worth summarizing why it’s arguably worth the effort in the first place.
Studies like these suggest that IT departments need to clarify their generative AI policies sooner rather than later, as a “wait and see” approach could risk falling behind.
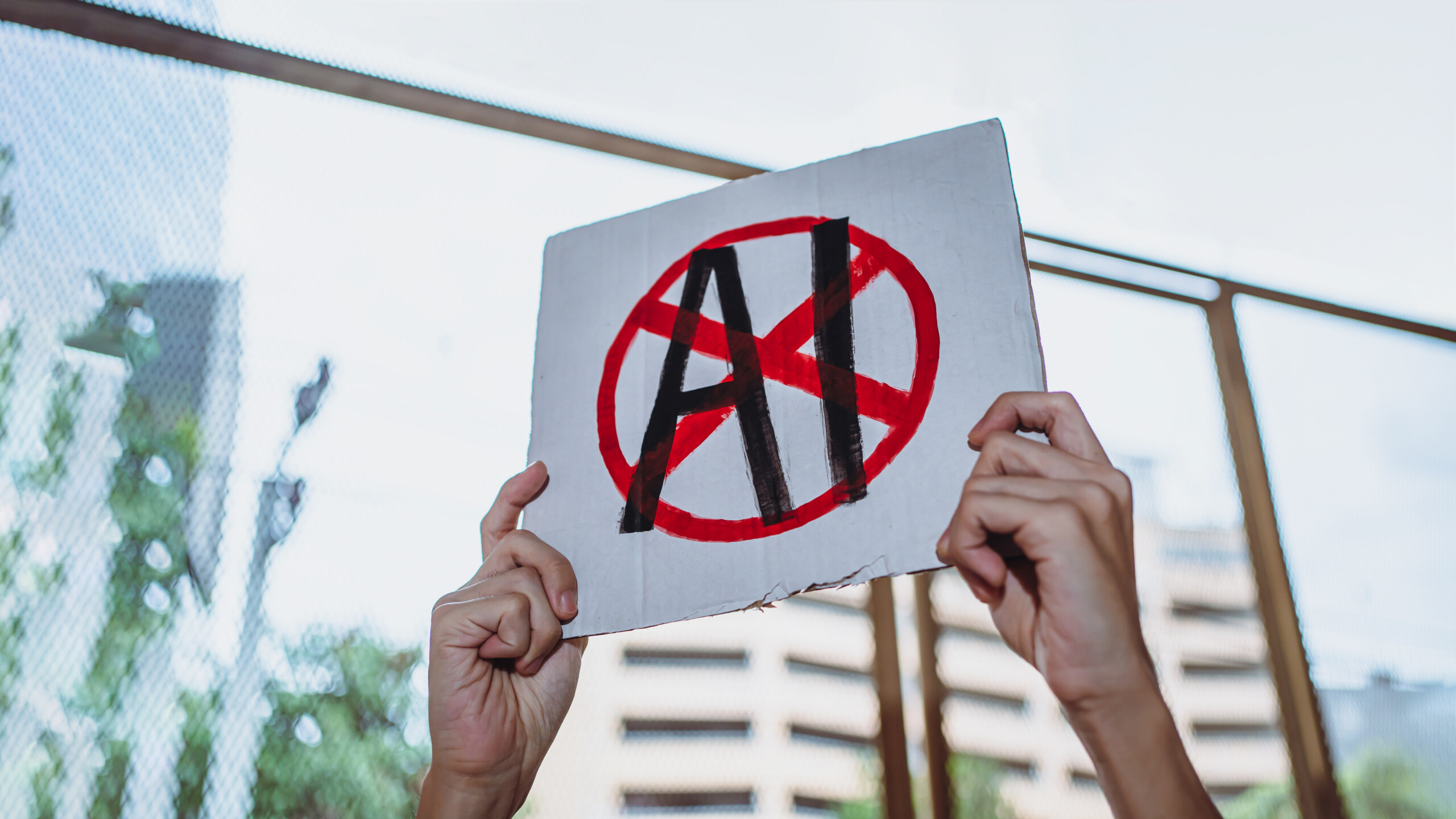
IT Policies for AI: From “Hard No” to “Anything Goes” (and Everything In Between)
When it comes to operationalizing generative AI in the workplace, there are a few different postures IT departments can take:
As for which stance makes the most sense – the answer is “it depends.” Let’s start by looking at a framework for assessing the benefits and risks for your specific organization.
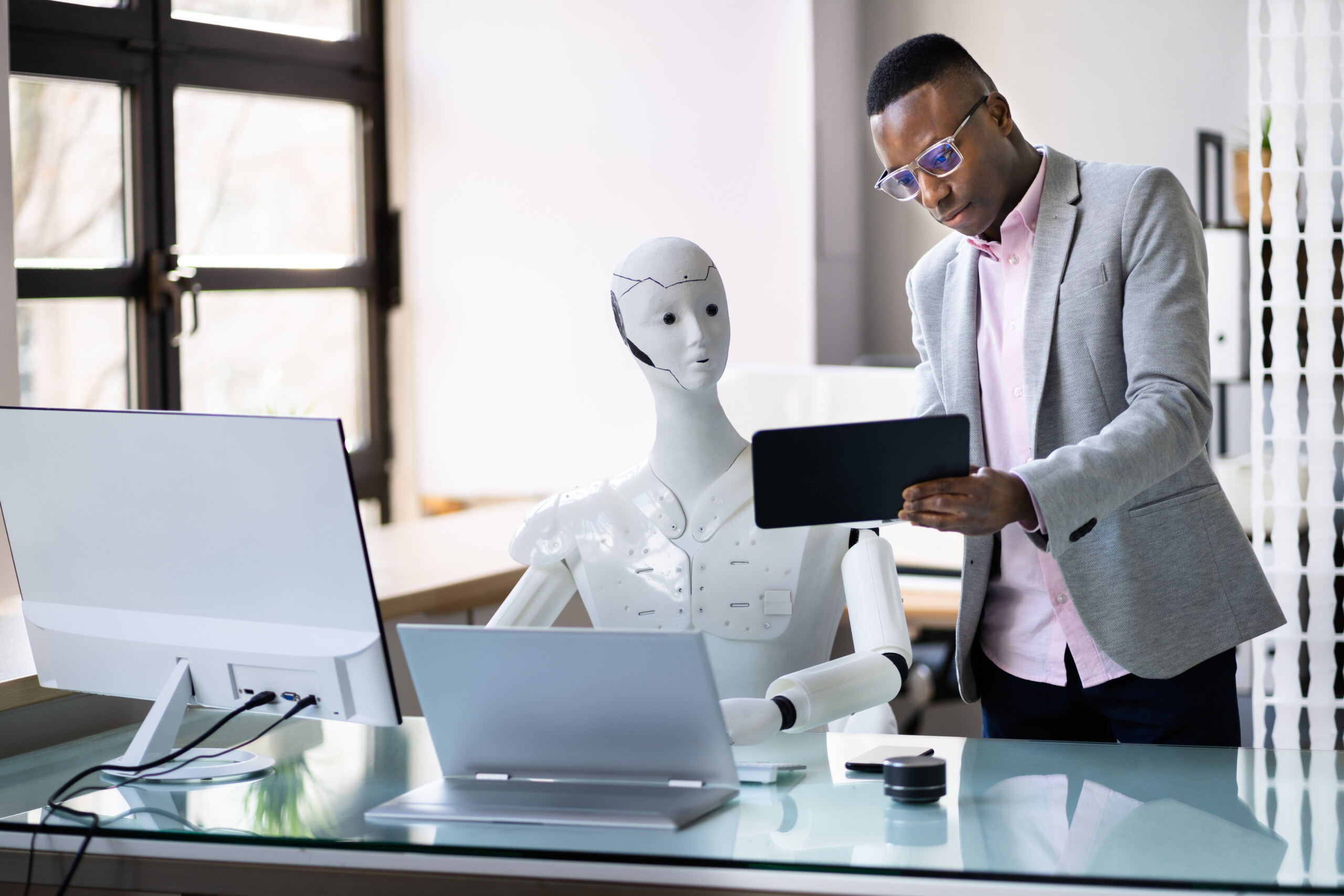
A Framework for Identifying & Assessing AI Risks
Deploying generative AI raises a number of considerations for IT, namely:
Control
The big fear around AI and enterprise / institutional data is that public AI models might use your proprietary information to answer other users’ questions. And this fear is not unfounded, given how some image-generating AIs recycle the work of popular illustrators and painters, sometimes even including the original artist’s signature in AI-generated output. Thus it’s not unimaginable that, if an engineer on your team is using their personal AI account to talk through a design problem, some of your innovations and trade secrets might get shared with a rival company’s engineers asking the AI similar questions.
That said, most of these issues only apply to free or cheap consumer-level AI accounts, where allowing the AI provider to train their model on your input essentially pays for your access, the way letting social media platforms use your personal data for marketing subsidizes your free profile.
Obviously, this rules out the “BYO” approach (i.e. letting people use personal AI accounts) for 90% of organizations. At the same time, while security might seem to favor the “Hardline” approach to AI (“just say no”), if we’re honest we know that workers probably will use cheap/free and insecure AI accounts if IT doesn’t provide sanctioned access.
Given that, wise IT leaders probably want to stay away from those two extremes and focus on the three middle approaches, instead – enterprise accounts, proxies, or (if warranted) building a proprietary model.
Cost
When it comes to cost there are two risks with most commercial AI platforms. First, employees might use it too much and costs will balloon (for the latest and greatest AI models like Chat GPT4 and Claude 3, the cost of “tokens” – a measure of usage roughly correlated to word count – can add up quickly). Second, people might not use it enough to justify the monthly subscriptions. And there’s also the question of how much of that usage is related to actual work versus people merely tinkering or having AI write their personal blog or do their MBA homework.
As for custom development, while it’s not impossible for an organization to build its own generative AI model, doing so would take a team of expert data scientists and software developers, around $1.5 million of rented processing power (at the low end), several trillion words of text to train the AI, and maybe about a year to do all the computing and quality assurance testing.
While this kind of moonshot project could make sense if you wanted your AI to do something incredibly strategic and lucrative (like Bloomberg pretraining an LLM specifically for financial expertise) for most organizations it’s a pointless exercise, going to all that effort just to end up with something that’s not quite as good as the commercial platforms for general purpose tasks.
Liability
Over the past few years, many articles have been written about how AI models have cited nonexistent court cases when used for legal research or spread medical misinformation or perpetuated racial bias. However, while the risks of a workplace AI account generating inaccurate or offensive information are real, they are also overreported in the same way as crashes involving driverless cars (despite the fact that driverless cars get in half as many accidents as humans per million miles.)
In reality, AI is far more likely to monitor and prevent hate speech than generate it, and many of the worst incidents are caused by human mischief-makers going to ridiculous lengths to trick AI models into saying offensive things.
This is another area where IT (and legal and HR) departments might be tempted to take a hard stance against AI: however it’s also an area where – if organizations ban AI – workers might use private, unmonitored accounts surreptitiously. By contrast, having workers go through training on responsible AI usage then assigning everyone an enterprise account or using a proxy to mediate and monitor AI usage might be the more pragmatic approach.
Also, while the process is too complex to get into here, organizations with extreme concerns about liability could also use enterprise-grade AI accounts and proxies to send conversation transcripts to a database for auditing, then use AI to screen those conversations in a “Who watches the watchers?” dynamic.
Purpose
Finally, there’s the question of what, exactly, people intend to do with generative AI. The truth is, not all tasks are equally suited for AI, not all jobs are likely to see immediate productivity gains from AI, and not all workers are equally adept at getting quality results from generative AI.
This has led to a growing cottage industry of AI proxies and copilots (e.g. Parrotbox.ai, Aiprm.com, or CustomGPT.ai) that give workers access to specific prompts, designed by professional prompt engineers for specific business purposes.
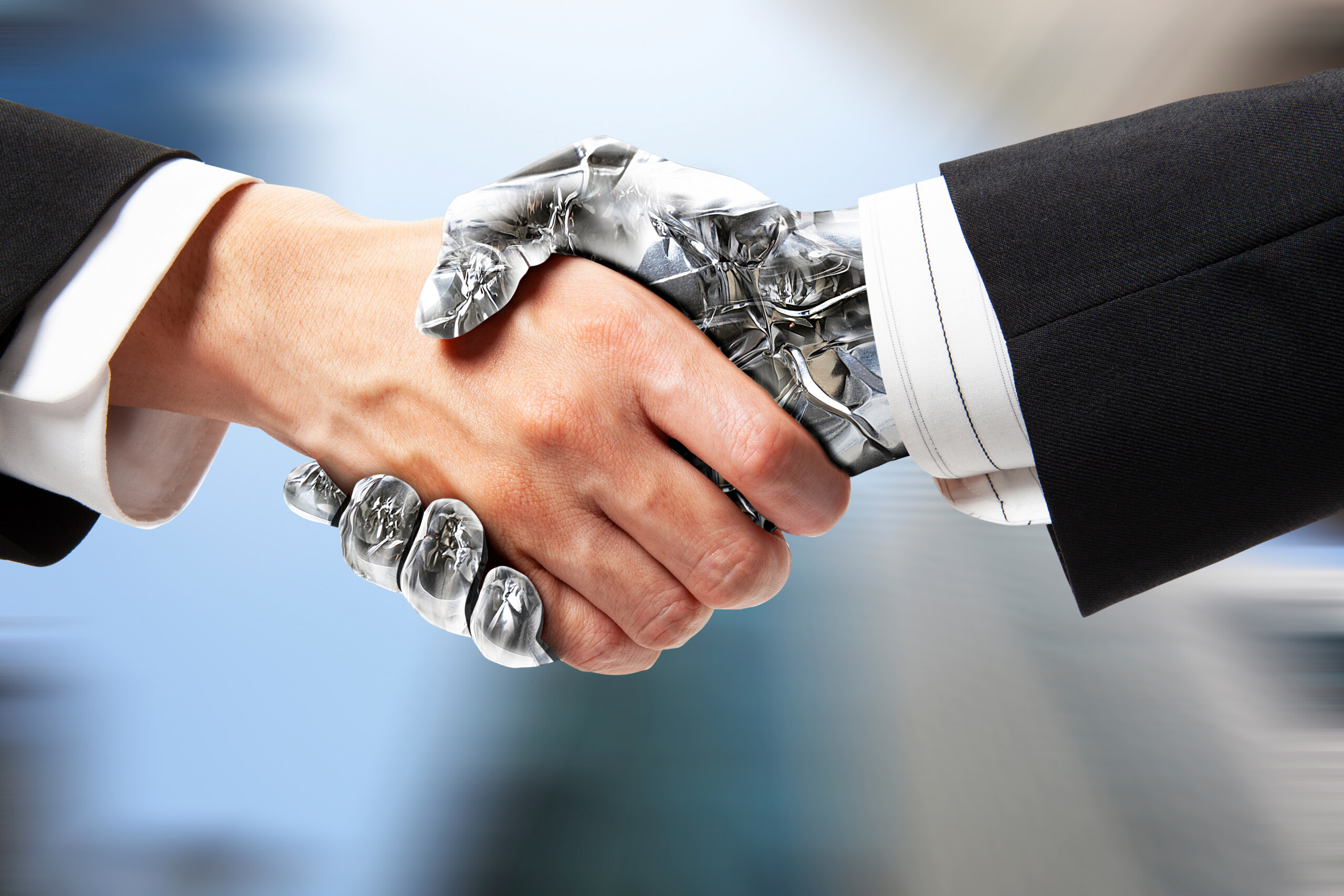
Selecting the Best Approach
With the framework above in mind, let’s revisit the policies discussed at the start of this article, and think through when each might be well-suited or poorly suited for a particular organization.
In the end, if you only believe half of the hype (or even a tenth of the hype) around generative AI, the potential benefits for organizations are massive. Investment firm Goldman Sachs believes two thirds of jobs will be at least partly automated through AI, and AI could increase global GDP by 7% while saving employers trillions. Meanwhile, employees don’t want to be left out of the AI loop: a D2L survey found 60% of workers want to use AI more often in their jobs, and a separate study by the Oliver Wyman Forum found 80% of workers want more training on how to use AI.
By some measures, the adoption rate for generative AI in the workplace is on course to match that of smartphones, the Internet, or even electricity. This places IT departments in a delicate position, between the mandate to support and safeguard their organizations while at the same time giving workers the tools they need to be productive.
However you ultimately decide to operationalize AI in the workplace, hopefully this article provided some useful food for thought, And if you need support with training or technical solutions for operationalizing AI in the workplace, feel free to reach out via https://www.sonatalarning.com/ai